Technological advancements have gone beyond expectations while merging the gap between humans and machines. Nowadays, machines can think like humans while offering realistic outcomes. Neuromorphic computing or neuromorphic engineering is one example of such machines that have human-like thinking abilities.
Over the years, neuromorphic engineering has evolved significantly, indicating cross-industry integration. In 2023, the global neuromorphic computing industry recorded a valuation of $92.45 million, which is about to reach $672.05 million by 2028. The progression in the AI and ML sectorsย has beenย a key element for such growth.ย ย
Let us understand neuromorphic engineering and computing more distinctly, alongside obtaining knowledge on how it works, its benefits, and challenges. Letโs dive in…ย
What is Neuromorphic Computing?
Neuromorphic computing is the future of computer engineering, which defines a method where computers have the ability to think and offer results like humans. In simple words, such a mechanism can imitate the human brain and thinking capacity. When a computer has a neuromorphic approach, both its software and hardware compatibly contribute to the process.ย
Though such technologies have recently received popularity with the emergence of AI and ML, they have been around for decades. After the invention and prominence of computers, several scientists and psychologists studied the human brain and the competency of computers.ย ย
Finally, in the 1980s, Misha Mahowald, an American psychologist, and Carver Mead, an American engineer, invented the very first silicon retina, neurons, and synapses. The invention led to the formation of neuromorphic engineering and computing.ย ย
How Does Neuromorphic Computing Work?ย
Neuromorphic engineering or computing is an event-based tactic that highly depends on machine learning and non-machine learning algorithms and methodologies such as deep learning, plasticity, graphs, reservoir computing, and evolutionary algorithms. Using these techniques, the software and hardware of computers attain the potential to mimic the human brain.ย
Neuromorphic hardware is often known as a spiking neural network (SNN) that evaluates and stores data or information similar to that of biological or human neurons. Another core element of neuromorphic engineering is artificial synaptic tools.ย ย
These tools aim to imitate human brains by transferring electrical indicators to spiking neurons with the assistance of analog circuits. Following the transfer, spiking neurons identify and encode the modifications in the analog signals.ย ย
Neuromorphic computing includes SpiNNaker and BrainScaleS tools. The former is operational for effective spike interaction, and the latter supports neurons and synaptic devices by mimicking analog electrical algorithms.ย ย
Advantages:ย
High Adaptability:ย
Neuromorphic computers are highly flexible and adaptive to solve diverse and complex problems. As such mechanisms intend to emulate the human brain, they depend on continuous real-time analytics and learning. This approach helps in evolving stimuli, where computers can easily identify challenges and address them in the most human-like way.ย ย
Unified Processing:ย ย
Like humans, neuromorphic computers can process and perform multiple tasks at once, processing data fromย severalย areas together. To accomplish such capability, neuromorphic computers assess and gather dataย collectivelyย from every neuron.ย ย
Power Efficiency:
Neuromorphic systems are energy efficient as they consume power for limited occasions. It follows an event-based approach, where neurons and synaptic devices operate together to transfer and decode data. In the process, solely the system spikes utilize energy.ย ย
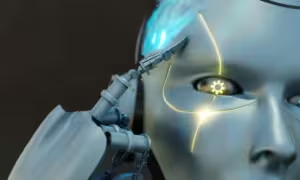
Challenges:ย ย
Confined accessibility: Since neuromorphic computing is still in the development phase, it is not commercially available and accessible to the common public. Therefore, the full competency of this mechanismย is not knownย yet.ย ย
Accuracy: Experts often question neuromorphic computersโ accuracy parameters as they are not available publicly. Though they are more advanced and include high costs, many developers find traditional computers more accurate than them.ย ย
Unclear standards:ย Being inaccessible to a larger number of audiences, there are no set standards and benchmarks of neuromorphic architecture. It has been a long-term challengeย inย the progression of the technologyย and its performance assessment sectors.
Complexity:ย Neuromorphic computers include complex algorithms and learning curves, amalgamating bioscience, math, neuroscience, computer science, and others. Such an approach is difficult to practically put in and drive beneficial outcomes.ย ย
Wrapping Up!ย ย
Neuromorphic computing is doubtlessly an evolving sector with promising advantages and unavoidable difficulties. This technology can be remarkably effective in domains such as cybersecurity, robotics, autonomous vehicles, and others. However, it is necessary to thoroughly evaluate its limitations.ย
Was the blog informative and value-added? If so, then check out our other in-depth resources.
Read More:
Understanding the Virtual Private Cloud: A Modern Approach to Cloud Computing